Towards Meaningful Ethics in Data Science
By Victor Zimmermann
This January I gave a short talk at PyData Berlin on best practices in ethical data collection. Behind that title, which in retrospect could have used some spicing-up, were a summary of three years’ worth of thinking, discussions and readings on ethics and how to incorporate and really cement them in a professional environment. As is abundantly obvious, I am not an ethicist. Still, I hope this write-up of the ideas that fed into that PyData talk will move one or two readers to reflect on their own role in their profession and seek out the people that really do know what they are talking about.
Also, I have privilege oozing from every pore of my body, being decidedly white and male-presenting. I have not experienced discrimination first-hand, and the algorithms deployed in the world today tend to favour people who look like me. Those who do not are systematically excluded from spaces where their concerns could be voiced. I aim to present these issues and ideas as authentically as possible, but that is not a replacement for listening to those affected by algorithmic decision making, the tech industry and toxic tech bro culture.
While I certainly have unqualified thoughts on other fields as well, I will try to stick to those on Data Ethics for this piece. That means I will not get into machine ethics (machines as moral actors, think self-driving cars), algorithmic bias on a technical level or bullshit (i.e., fake news).
I have little interest in giving out concrete moral assessments on any particular issue in Data Science, that is not what is at the heart of Ethics and it is not bringing us closer to any meaningful change in how this profession operates. Rather we ought to take a bird’s eye view on what ethical safeguards are already tried and tested across the industry, how they work, what assumptions they operate on, and if those assumptions hold up.
A workable definition of ethics can be taken from Petra Grimm, et al. who write:
“[Ethics] invites us to think about what kind of people we want to be. […] But we also have to learn how to live with the consequences of deciding wrong.”
In other words, to what standard are we to hold our own profession, and what happens if we cannot live up to that standard?
Apart from the fact that we have to look ourselves in the mirror every morning, there are also corporate interests which must be considered. Ideally, those enable actual ethical behaviour of the company, but not necessarily. According to most big tech companies, data science is a shadowy craft that is best not undertaken in broad daylight, ideally done far away from public scrutiny and government oversight. A good shitstorm can halt any project and government red tape has brought many enterprises to a stand-still. That is why every dotcom with a 027-zip code has a dedicated ethics team, to show that they can very well regulate themselves, thank you, nothing to see here. Of course, who acts morally most often than not also looks moral, but the reverse might not be the case. Keep that distinction on mind.
Conveniently, the common approaches to Data Ethics also roughly translate to the major schools of Ethics theory: Teleological ethics, deontological ethics, and virtue ethics. We will go through each of them in order.
A central problem, I would argue the central problem of Data Science is the inherent bias in all data. The important distinction is not between biased and unbiased data, but between wanted and unwanted bias. And while the wanted biases are often hard to crack, machine learning algorithms exceedingly excel at finding those unwanted biases, however hidden, by proxy or in plain sight, and reproduce them at every step down the line.
If we take those bias amplifying algorithms as a black box, there are two places left one can try to mitigate biased outcomes. The data collection and the application. What kind of data do we feed the algorithm and what damage can it do? One landmark paper by Timnit Gebru tackles both questions.
In Data Sheets for Data Sets Gebru argues for deliberate documentation of the entire data collection process. Akin to spec sheets for electronic components, those data sheets should come with every data set. This has two functions: Whoever uses the data set next has the best approximation of what information, concerns and problems the team assembling the data had, and can act accordingly. Second, the data collecting team is guided by the questions in the data sheet to engage in ethical decisions and considerations themselves when collecting their data. They are asked about any conflicts of interest, about the demographic make-up of potential subjects, about any privacy concerns they might have. They are also asked to what tasks the data should not be applied to, and why.
Teleological ethics deals with objectives and purposes, impact assessments, costs and benefits. Utilitarianism comes to mind. Data sheets enable you as a data scientist to make more informed ethical decisions, without taking away any agency. If your decision making is based on the costs and benefits to those affected, data sheets are probably the best approximation you can ask for.
Most of us deal with fundamentally human data. As a computational linguist, human data points are all I have. When looking at other professions in similar situations, a common theme emerges. Take medicine, for example. After the horrific acts of the Nazis during the Second World War, multiple questions needed urgent answers to prevent any such acts to happen again in the future. What kind of red lines are there? How are they enforced? How do we as a profession want to be perceived? Based on the Hippocratic Oath, the Declaration of Geneva was written in 1946 and revised at numerous times during its history and is still one of the strongest ethical codes of any profession to this day. Through it, every practitioner pledges to uphold the dignity of a human life, respect for the patient, whoever they may be, and a responsibility towards the wider community.
In reviewing several ethics codes across multiple professions, Jacob Metcalf defines seven outward facing goals, which deal with public perception and responsibility, and nine inward facing goals that establish internal review and reform processes.
Ethics codes provide a straightforward response to our two demands for ethics outlined at the top. They are both aspirational in their goals and consequential through their codification. The severity of punishment for breaking a code depends on social context and the profession in question, but even breaking the notoriously toothless journalistic code of ethics often leads to industry-wide ostracism.
Ethics codes reflect deontological ethics, they enshrine duties and rights and project intrinsic moral values. By upholding and practicing a code based on these moral values, duties and rights are guaranteed for those practicing and those affected by the profession, so the theory.
Still, already established codes of the medical or journalistic profession are taught throughout medical school and college, they are known by most prospective professionals even before the first class starts. You know what a good doctor looks like, but a good data scientist is far less easy to define.
I would argue a good doctor, a kind, respectful, caring doctor is not all those things because of a strict ethical code, but because that is the expectation of them. Because their ideal doctors are kind, respectful and caring, they also aspire uphold these virtues. That is not to say strong oversight and a rigid code of ethics does not protect patients, but as a profession we should not only try to find a baseline of acceptable behaviour but strive towards being actually virtuous practitioners.
But how do we get there? What even are the virtues a data scientist should live up to? Luciano Floridi and Josh Cowls of the Oxford Internet Institute propose five core principles for AI in society, namely beneficence (Does it promote human well-being?), non-maleficence (Does it inflict harm?), autonomy (Does it impair or enhance human decision making?), justice (Is it fair?) and intelligibility. The first four are common principles from bioethics, the fifth, explicability, is meant as an umbrella term for intelligibility (How does it work?) and accountability (Who is responsible if it does not?).
But even with a firm set of guiding principles, we are not yet any better for it, or as Morley, et al. put it:
“The gap between principles and practice is large.”
One approach to practical virtue ethics is called embedded ethics, which largely deviates from the way data ethics is taught in computer science courses around the world. The common practice is to look at cases of malpractice or known issues and work through papers that analyse and measure unfair algorithms or provide mitigation techniques to improve these algorithms. They do not train data scientists to actually become aware and possibly spot the next scandal before it happens, but only to respond to past wrongs. By looking at the major scandals, the big issues, we are still somewhat blind towards all the ills and woes that we have yet to come across. With such a fast changing field as data science, there is little reason that future challenges will just be iterations on the ones we have seen before and it is naïve to assume that we have seen it all.
In contrast to the underfunded and auxiliary ethics courses mentioned above, embedded ethics aims to be a core component of every course taken during a data scientist’s education, just as ethics should be a core component of daily practice. Assignments should include questions about ethical implicatures, students should be encouraged to share their code and contribute to open source projects, every algorithm should not only be discussed in terms of use-cases, but also misuse-cases.
The analogy to learning an instrument becomes rather obvious. By practicing playing the violin, the music gets better, but you also become a better violinist in the process. And just like learning a new language, practicing ethics becomes a lot harder when not in a school environment.
That is why role models are so important, they provide guidance and a tangible target to aim for every day. Data science may not have that many obvious role models, but they are there if you know where to look. I provide a list of researchers and activists that inspire me at the end, but that may not reflect what you are after. By engaging in critical data science, by joining Data for Good and open source projects you can come across tons of interesting and passionate people that inspire you every day.
Data sheets and ethics codes are important to hold organisations and individuals accountable and to higher standards, but actually being better takes practice, effort and motivation. But so takes learning a new language and that should not stop anyone from doing so either.
Author:
Victor Zimmermann -
Junior Consultant
Computational Linguistics
Speaker at PyCon.DE 2019 & PyData Berlin 2021
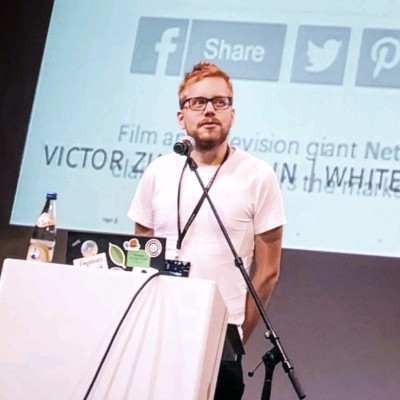
Sources:
Mitchell, M., Baker, D., Moorosi, N., Denton, E., Hutchinson, B., Hanna, A., Gebru, T., & Morgenstern, J. (2020). Diversity and Inclusion Metrics in Subset Selection. In Proceedings of the AAAI/ACM Conference on AI, Ethics, and Society (pp. 117–123). Association for Computing Machinery.
Timnit Gebru, Jamie Morgenstern, Briana Vecchione, Jennifer Wortman Vaughan, Hanna Wallach, Hal Daumé III au2, & Kate Crawford. (2020). Datasheets for Datasets.
Facca, L. (2020). Exploring the ethical issues in research using digital data collection strategies with minors: A scoping review. PLOS ONE, 15(8), 1-17.
Bergstrom, C., & West, J. (2020). Calling bullshit: the art of skepticism in a data-driven world. Random House.
Misselhorn, C. (2018). Grundfragen der Maschinenethik. Reclam.
Grimm, Petra and Keber, Tobias O. and Zöllner, Oliver (2019). Digitale Ethik: Leben in vernetzten Welten. Reclam.
Bender, E., & Friedman, B. (2018). Data statements for natural language processing: Toward mitigating system bias and enabling better science. Transactions of the Association for Computational Linguistics, 6, 587–604.
Metcalf, J. (2014). Ethics codes: History, context, and challenges. Council for Big Data, Ethics, and Society.
Floridi, L., & Cowls, J. (2019). A unified framework of five principles for AI in society. Harvard Data Science Review, 1(1).
Morley, J., Floridi, L., Kinsey, L., & Elhalal, A. (2019). From what to how. An overview of AI ethics tools, methods and research to translate principles into practices. arXiv preprint arXiv:1905.06876.
Bezuidenhout, L., & Ratti, E. (2020). What does it mean to embed ethics in data science? An integrative approach based on the microethics and virtues. AI & SOCIETY, 1–15.
Photo:
https://unsplash.com/